Latent Dirichlet Allocation
Latent Dirichlet Allocation (LDA) is an unsupervised topic modeling method used to determine which words are more probabilistically associated with a given number of unspecified topics. Like clustering and ARM, topic modeling can be used to cluster text data and explore terms commonly found together within documents. LDA is an iterative algorithm based on the dirichlet distribution. The number of topics is given by the user and the output consists of lists of terms that are likely to occur within each ambiguous topic.
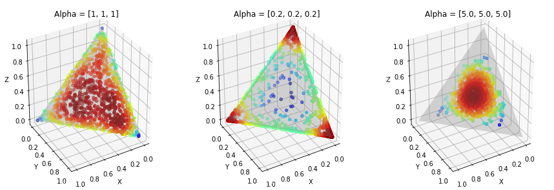
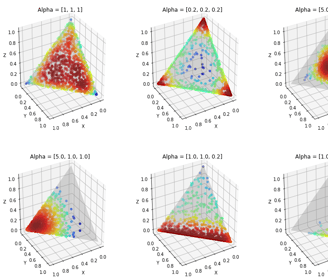
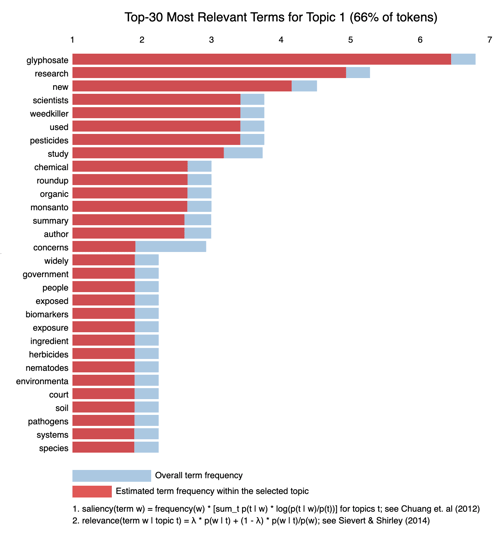
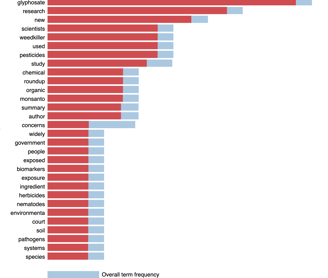
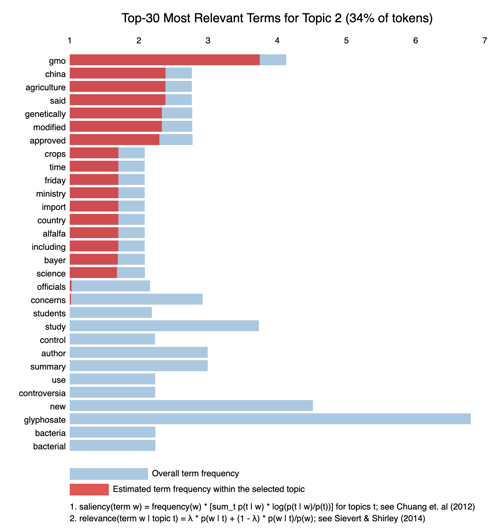
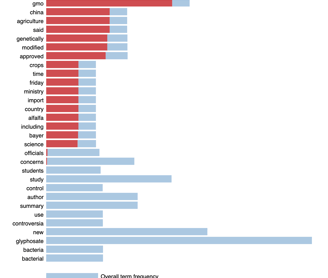
In the context of the GMO debate, LDA can be used to explore the difference in topics within news covering two major implementations of genetic modification within crops. Glyphosate is a strong growth-inhibiting chemical found in most weed killers. Herbicide resistant crops are engineered to withstand harsh chemicals such as glyphosate in order to allow for the use of pesticides in farming without damaging the crop itself. These herbicide resistant crops represent a significant use case for genetic modification in crops. On the other hand, another significant genre of GM crops is BT crops. "BT" represents the Bacillus Thuringiensis protein which is particularly harmful to the digestive tracts of insects. Therefore, incorporating this protein into crop DNA provides plants with natural replant against predatory insects. In this way, the crop is protected without the use of topical pesticides.
Because these two use-cases have different side effects, the pros and cons debated tend to be different as well. For this reason, it is assumed that the narratives and discussions surrounding these subjects would differ. For this exploration data was gathered from NewsAPI.org articles under two keyword searches: